With the explosive growth in the technologies where people and businesses have started leveraging the digital platforms, automotive industries have very much evolved from the traditional ways. Means of interactions between driver & passengers, way of vehicles connectivity with the outside world have changed which creates the demand for faster and cheaper data processing. In today’s demanding world, the automotive industry is one of the fastest-growing industries and Edge Computing is playing a key enabling role in transforming the things around the automotive space. Exponential data growth in connected and autonomous vehicles and real-time data handling along with new technologies is driving Edge Computing. Emerging real-time applications in the automotive industry such as video analytics, self-driving vehicles, driver monitoring systems, surveillance cameras, artificial intelligence algorithms continue to accelerate the edge computing systems.
What is Edge Computing?
Digitization is creating a need for real-time data processing. Edge Computing enables to process or compute data at the place where it is required or where the data is generated or at the “Edge”. In layman terms, Edge Computing brings data and computation closer to the edge where people or devices consume that information rather than doing it in a centralized manner. This is done to maintain real-time results without affecting any application performance and latency issues.
In an earlier time before the rise of Edge Computing, data collected from any device or system was sent across to the common server where various computing algorithms like aggregation, analytics used to run or we can say computing happens on a centralized platform with no real-time results. This is acceptable only for data analytics and applications that are not time-critical however time constraint applications demand faster data processing which generates the need for Edge Computing.
Need for Edge Computing
Instantaneous Reliable Decision – Edge Computing helps in getting real-time results at the edge and for the analysis of vast amount of data generated through connected and autonomous vehicles where an instantaneous reliable and crucial decision is required.
Better Performance – Having real-time results and computations at the edge or where the information needs to be consumed leads to get faster data processing and better network performance.
Below is the general block diagram of typical Edge Computing solutions
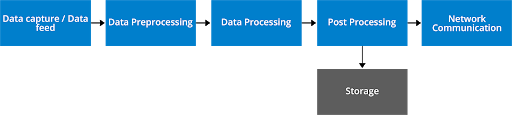
Stage 1: Data capture / Data feed
This can be output from any camera or data from any source
Stage 2: Data Preprocessing
This is required to align incoming data to the format as expected by the AI algorithm
Stage 3: Data processing
This can be done using a GPU or any Accelerated Analytics Engine. Depending on the load and concurrency, required HW acceleration needs to be chosen
Stage 4: Post-processing
This involves any action that has to be done based on Inference output
Stage 5: Data storage
This involves inferred data to be stored in the device for future reference
Stage 6: Network communication
This is usually done when cloud communication is required and any events have to be updated
Edge Computing in the Automotive Industry
With the ever-evolving technologies and rise in connected and autonomous vehicles in the automotive space, challenges like delay in data processing, network performance, data transfer in the connected vehicles, real-time results, on the spot reliable & crucial decisions, processing of vast amounts of data, etc. have also been increasing. In order to address these challenges focus on leveraging Edge Computing is more as compared to other computational methods. Let’s take an example of an Autonomous vehicle, a vehicle running on a highway sees an obstacle so it has to apply brake instantaneously. Here, sending the live video to the cloud and then waiting for cloud triggering to apply brakes can create latency in the results. With the help of Edge Computing, the live video can be processed faster within the device that captured it and real-time action can be taken without any adverse effect. Video analytics at the edge is transforming the automotive industry. Let’s discuss how we can have benefited from Edge Computing in the automotive sector.
Below are some of the possible use cases where Edge Computing plays a vital role
Rash Driving Detection
With the help of Edge Computing, data collected from the vehicle’s Accelerometer and Gyrometer can be computed at the same place where it is gathered and it generates the corresponding required alerts to maintain the safe driving standards. Data also monitored at a high sampling rate in order to detect any sudden acceleration, instantaneous braking, car crashes, need of airbags, etc.
Vehicle Health Monitoring
Vehicle health monitoring is one of the applications driving Edge Computing systems. Here, On-board diagnostics tools and interfaces in the vehicle help in detecting the real-time problems and generates the required alerts for the drivers. Data received from the onboard interface can be continuously monitored with the help of Edge Computing and any failure or risk can be predicted before actual damage.
Driver Monitoring Systems
Driver monitoring system (DMS) ensures safe driving standards by alerting the driver if he falls asleep or gets distracted while driving. It also creates various warnings based on real-time situations such as seat belt absence, signal violation, lane violation and safe distance with front side vehicles.
Factors Contributed for Trending of Edge Computing
Drastic growth in On-chip capabilities
Even though the need for Edge Computing has become unavoidable, however, it is not practically possible without On-chip support. Due to recent technological advancements in VLSI and Power electronics, the capabilities of chips have drastically improved. The way mobile phones have changed in the last 10 years is an example.
Reliability
The major advantage with Edge Computing is – Reliability. Data capture, Data processing and Actions are done within the same device or at the “Edge” that accelerates real-time decision resulting in instantaneous actions. This reduces the dependencies or no dependencies with external factors like network stability, weather, interferences, etc.
Deciding factor for Success
This is one important trade-off factor to be considered while designing the Edge Computing requirement. As we add more on-chip capabilities, they require more power to carry out all functionalities. Many chip manufacturers have their Edge Computing solutions however only the manufacturers having efficient computing based on performance and power consumption, will have upper hand in the competition.
How does VVDN help OEMs?
VVDN leverages its expertise in product engineering, manufacturing, cloud & applications, IoT, edge computing, AI/ML, deep learning to deliver various AI-based automotive solutions such as ADAS solutions, Driver Monitoring System with inbuilt machine learning algorithms in order to generate various safety warnings & alerts including drowsiness detection, mobile phone usage detection, number plate reading, safe distance following, Side mirror replacement, etc. VVDN with its reusable frameworks and edge computing capabilities addresses the OEMs concern for fully reliable, scalable, and efficient automotive solutions with accelerated time to market.
Click here to read more about VVDN’s Vision expertise or for any such requirement feel free to contact: info@vvdntech.com